The industrial revolution of the 21st century is not being driven by steam engines or assembly lines but by algorithms. Artificial intelligence (AI), once confined to the realm of speculative fiction, is now at the heart of a new manufacturing renaissance. From precision-driven processes to predictive maintenance, AI promises to transform the factory floor into an ecosystem of data-driven efficiency. Yet, despite its potential, AI adoption in manufacturing has remained curiously tepid, hindered by challenges that are as old as technology itself: cost, uncertainty, and fear of the unknown.
At a recent panel discussion during Tech and AI Live, industry leaders from Microsoft, SAP, and Samsung came together to explore the opportunities and hurdles in adopting AI within manufacturing. Their insights underscored not only the vast potential of this technology but also the critical steps needed to realize its promise fully.
AI’s Role as an Enabler of Precision and Productivity
Jeff Winter, a manufacturing industry executive at Microsoft, described AI as a "decision-making tool" that supplements human intelligence. Unlike traditional machinery, which follows predefined instructions, AI has the capacity to analyze vast quantities of data, identify patterns, and make informed recommendations. This makes it particularly suited for two primary functions: augmentation and automation.
In its augmentative role, AI enhances human decision-making by offering predictive insights and scenario simulations, which are invaluable in a manufacturing environment where every second counts. Whether determining the optimal supply chain strategy or forecasting equipment failures, AI’s ability to sift through reams of data far exceeds human capacity. Automation, on the other hand, represents the next frontier—entrusting AI with decisions that previously required human oversight. Self-driving vehicles or autonomous assembly lines are prime examples of automation’s potential, although trust in these systems remains a work in progress.
But while AI excels in analyzing structured datasets and performing repetitive tasks with unerring precision, its limitations are equally striking. It lacks the "common sense" and contextual awareness that allow humans to adapt to new and unforeseen scenarios. This cognitive gap is why Winter advocates for a cautious yet strategic approach to implementing AI, recognizing both its strengths and its limitations.
Use-Case: AI driven On-Demand Manufacturing
One area where AI has already delivered tangible results is in on-demand manufacturing, a model that has revolutionized the production and procurement of machined parts. By integrating AI with digital manufacturing platforms, companies can now offer buyers unprecedented speed, cost efficiency, and quality.
A leading example of this innovation is InstaWerk, which leverages AI to analyze technical drawings and CAD models in a fully automated manner. The system extracts critical details such as tolerances, materials, and other specifications directly from the technical drawings. It then cross-references this information with a 3D-analysis of the CAD-model, enabling a comprehensive understanding of the manufacturing requirements. Using this data, InstaWerk instantly generates a price, detailing not only the price but also the most efficient machining process to achieve the desired specifications. This level of automation ensures that buyers receive precise, customized solutions within minutes, rather than waiting days for traditional quoting processes.
For buyers, the advantages are transformative. AI-powered platforms like InstaWerk analyze customer designs and automatically generate optimal instant quotes and machining strategies, reducing the lead time from weeks to mere days. This is particularly advantageous for industries like aerospace and automotive, where precision and speed are paramount. The cost savings are equally compelling: by automating much of the quoting and logistics process, these platforms can offer competitive pricing for CNC-Milling or CNC-Turning without compromising on quality.
Quality, often a concern in high-precision manufacturing, is bolstered by AI’s ability to ensure consistency. Machine learning algorithms monitor production and reclamation rates, identifying low-performing suppliers and excluding them from the network. For buyers of CNC-machined parts, this means fewer rejected parts and it translates to greater reliability. On-demand manufacturing, powered by AI, exemplifies how technology can democratize access to high-quality manufacturing while reducing waste and inefficiencies.
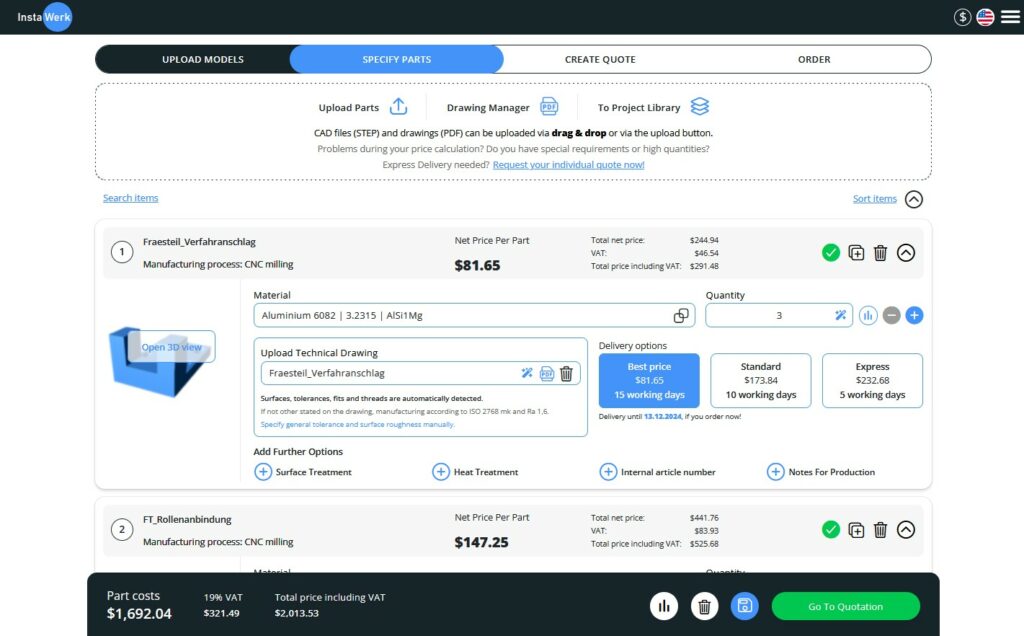
Predictive Maintenance and Quality Assurance
The integration of AI in predictive maintenance offers another compelling example of its transformative potential. Historically, maintenance strategies relied on fixed schedules or reactive measures, both of which are costly and inefficient. With AI, manufacturers can analyze sensor data—such as temperature and vibration patterns—to predict when a machine is likely to fail. This allows maintenance teams to intervene proactively, reducing downtime and extending equipment lifespan.
Similarly, AI has revolutionized quality assurance processes. Andreas Welsh, Vice President at SAP, highlighted how computer vision is being used to automate defect detection. By analyzing images of manufactured parts, AI can identify imperfections with far greater accuracy than human inspectors, ensuring that only parts meeting stringent quality standards reach the market.
But the implications extend beyond the factory floor. Demand sensing, another AI-driven capability, allows manufacturers to forecast future needs with remarkable accuracy. By integrating data from ERP systems, IoT devices, and external factors like market trends, manufacturers can optimize production schedules and inventory management, reducing overstock and minimizing delays.
Challenges on the Road to Adoption
Despite these breakthroughs, the journey toward widespread AI adoption in manufacturing is fraught with obstacles. Patrick Banger, Vice President of AI at Samsung, compared AI to an intern—capable of remarkable insights but only after extensive training. The analogy underscores one of the most significant barriers to adoption: the availability of high-quality data.
For AI to perform well, it requires vast amounts of labeled data, particularly for tasks involving rare events like machine failures. Collecting and curating this data is both time-consuming and expensive, posing a challenge for manufacturers already operating on thin margins. Additionally, the financial uncertainty surrounding AI projects—where benefits are often speculative—makes them a hard sell to risk-averse decision-makers.
Another hurdle lies in the human element. Implementing AI often necessitates a cultural shift, requiring employees to adapt to new workflows and managers to embrace a technology they may not fully understand. Overcoming this resistance will require not only technical training but also a concerted effort to demystify AI and build trust in its capabilities.
The Ethical and Transparent Future of AI
As AI becomes more ubiquitous, ethical considerations will take center stage. Autonomous systems, whether in manufacturing or healthcare, must be designed with fairness and transparency in mind. Banger stressed the importance of explainability, noting that AI systems must not only deliver accurate results but also justify their decisions in ways that are comprehensible to human users. This is particularly critical in high-stakes industries, where trust in AI could mean the difference between adoption and rejection.
Looking ahead, the future of AI in manufacturing will likely be defined by its ability to integrate seamlessly with existing systems. Welsh suggested that manufacturers should start small, leveraging AI features embedded in current software platforms. By doing so, they can reap immediate benefits while building the organizational capacity to tackle more ambitious AI projects.
Conclusion
The promise of AI in manufacturing is as vast as the challenges it seeks to address. From on-demand manufacturing to predictive maintenance, AI offers a roadmap to a future where efficiency, quality, and speed coexist in perfect harmony. Yet, realizing this vision will require a concerted effort to overcome data limitations, financial uncertainty, and cultural resistance.
For manufacturers willing to take the leap, the rewards are immense. AI is not merely a tool for optimization—it is a catalyst for innovation, capable of reshaping how we build, produce, and deliver in an increasingly complex world. The question is not whether manufacturers should invest in AI, but how quickly they can afford not to.